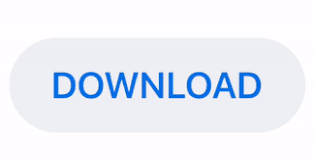

Jazmin Zatarain Salazar-Assistant Professor, TU Delftīernardo Trindade – Senior Optimization Engineer, Suez WaterĪntonia Hadjimichael – Assistant Professor, Penn State Thomas Wild- Assistant Research Professor, University of Maryland & Research Scientist, Pacific Northwest National Laboratory Julianne Quinn-Assistant Professor, University of VirginiaĬharles Rouge-Lecturer, University of Sheffield Jon Lamontagne-Assistant Professor, Tufts University Riddhi Singh-Assistant Professor, Indian Institute of Technology Bombay Joe Kasprzyk – Associate Professor, CU Boulder Below are two examples, the first is the autocorrelation function of a set of observed temperature values in Des Moines Iowa, the second is autocorrelation function of the temperature values at the same location as modeled by the MM5I regional climate model: The command generates a plot of the autocorrelation function. % T is your data set and k is the number of lags you would like to compute You can generate such plots in Matlab using the simple command shown below: autocorr(T,k) Plotting the autocorrelation graphically can be a helpful tool for quickly assessing the presence of autocorrelation within a data set. The calculation of autocorrelation for a number of different lags at once is known as the autocorrelation function. Should a data set prove to be strongly persistent, such methods will likely yield inaccurate results.Īutocorrelation is commonly computed by making a copy of the original data set, shifting the copy k points forward (where k is the lag over which you would like to compute the autocorrelation) and computing the Pearson correlation coefficient between the original data set and the copy. Calculating autocorrelation within a sample data set can also be a helpful for assessing the applicability of classical statistical methods requiring independence of data points within a sample. Temporal autocorrelation is also a fundamental concept for synthetic weather generation (for more detail see Julie’s fantastic series of blog posts on synthetic weather generation here). In atmospheric science temporal autocorrelation can be a helpful tool for model evaluation. Autocorrelation is a measure of persistence within a data set, which can be defined as the tendency for successive data points to be similar (Wilks, 2011).
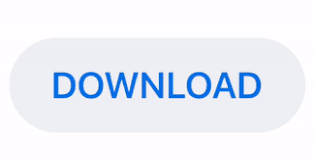